Clinical evaluation of RTOG-atlas based contouring, MRI-atlas auto-contouring, and neuroradiologist contouring of the hippocampi for hippocampal avoidance whole-brain radiotherapy
Introduction
It is estimated that 30% of all cancer patients will develop brain metastases with the rate of this likely to continue to increase with the ongoing improvements in systemic treatments in the palliative setting (1). Recent randomized evidence has now shown the treatment of multiple brain metastases to allow for better quality of life and memory outcomes when patients are given hippocampal-avoidance whole brain radiotherapy (HA-WBRT) with memantine as opposed to WBRT with memantine via simple opposed lateral photons (2,3). However, the workflow for this new standard is much more time-consuming, requiring inverse planning often delivered with arc therapy, fusion of a diagnostic or simulation MRI, and contouring of additional at-risk structures including the hippocampi. Accurate delineation of these organs-at-risk (OARs) is essential, especially of the hippocampi as these are the main avoidance areas for memory preservation. This is a time-consuming task requiring clinician expertise. Thus auto-contouring methods have been trialed with the aim to improve efficiency of workflow and reduce resources (4).
Auto-contouring algorithms are divided into two methods for OAR delineation – atlas based versus deep-learning. Most commercial radiation therapy software utilizes an atlas-based method in which the patient images are aligned and compared to the template images within a library of expert contoured OARs, or atlas (5,6). The selected template contours are then deformed and generated to match the anatomical structure of the simulated patient. Variation as to the quality of auto-contours will depend on the number and quality of templates in the atlas, the number of atlases used for auto-contouring, the selection of the most appropriate atlas, the image-registration techniques, the deformation accuracy, and contour post-processing (6-12).
This study was to evaluate the accuracy and potential clinical utility of hippocampal contours generated via the atlas-based Elements TPS versus clinician manual contours and how these compared to the contours of a neuroradiologist for HA-WBRT. We present the following article in accordance with the STROBE reporting checklist (available at https://dx.doi.org/10.21037/tro-21-15).
Methods
Ten consecutive patient datasets in which the whole brain was CT scanned with IV contrast and same-day MRI fusion were identified. The patients were deidentified and the hippocampi were contoured by three radiation oncologists following the RTOG atlas (13). A neuroradiologist also contoured the hippocampi on the same datasets. This study was conducted in accordance with the Declaration of Helsinki (as revised in 2013) and reviewed and approved by the Sydney Local Health District – Royal Prince Alfred Ethics Review Committee (reference: X20-0304 & 2020/ETH01446). Informed consent was not required as no patient information was attached to the MRI nor was any patient contact or information otherwise required for the purposes of this study.
All manual contouring was performed in Eclipse™ v15.6 (Varian Medical Systems, Palo Alto, CA) on CT/MRI fused data sets. The RTOG atlas included in the RTOG 0933 trial was used to assist in manual contouring. Automatic contouring was performed in the Elements V1.5 (Brainlab AG, Feldkirchen, Germany) treatment planning software in its ‘Anatomical Contouring’ workspace.
Statistical analysis
The resultant manual and automatic contours were compared by using a similarity coefficient metric (Dice similarity coefficient) and surface distance metrics (maximum and mean Hausdorff distance) in the Velocity V4.1 (Varian, Palo Alto, CA, USA) software.
Inter-clinician conformality was assessed using the Dice co-efficient for overlap and Hausdorff maximal and mean distances for variability. Auto-contours were generated for the same 10 patient datasets in Elements TPS and compared against a random oncologist contour using the same statistical methods. The RTOG-based clinician and MRI-atlas based Elements auto-contours were then compared to those of a neuroradiologist’s. The statistical significance between the intercomparisons was assessed using the Student’s t-test.
Results
The manual contours by the radiation oncologists had reasonable conformality with each other with an average Dice co-efficient of 0.766 for both the left and right hippocampi (Figure 1). Hausdorff maximum distance was 4.8 mm for the left hippocampus and 5.2 mm for the right hippocampus (Table 1).
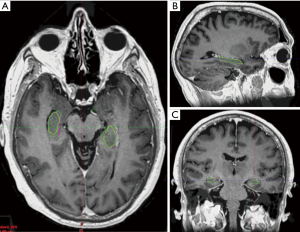
Table 1
Left hippocampus | Right hippocampus | ||||||
---|---|---|---|---|---|---|---|
Dice co-efficient | Hausdorff | Dice co-efficient | Hausdorff | ||||
Max (mm) | Mean (mm) | Max (mm) | Mean (mm) | ||||
RO1 vs. RO2 | 0.719 | 5.679 | 0.867 | 0.731 | 6.088 | 0.843 | |
RO1 vs. RO3 | 0.814 | 3.157 | 0.541 | 0.810 | 3.190 | 0.534 | |
RO2 vs. RO3 | 0.764 | 5.436 | 0.749 | 0.756 | 6.227 | 0.785 | |
Average | 0.766 | 4.757 | 0.719 | 0.766 | 5.168 | 0.721 |
When comparing Elements auto-contours with clinician contours, there was less spatial overlap with a lower average Dice co-efficient of 0.523 for the left hippocampus and 0.560 for the right (Figure 2). Average maximum Hausdorff distance was almost double that between clinicians at 9.213 mm for the left and 9.855 mm for the right hippocampus (Table 2).
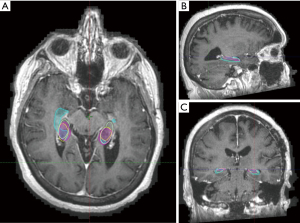
Table 2
Left hippocampus | Right hippocampus | ||||||
---|---|---|---|---|---|---|---|
Dice co-efficient | Hausdorff | Dice co-efficient | Hausdorff | ||||
Max (mm) | Mean (mm) | Max (mm) | Mean (mm) | ||||
RO1 vs. elements | 0.490 | 9.133 | 1.771 | 0.530 | 9.934 | 1.585 | |
RO2 vs. elements | 0.579 | 9.298 | 1.501 | 0.620 | 8.347 | 1.300 | |
RO3 vs. elements | 0.543 | 8.615 | 1.570 | 0.573 | 9.796 | 1.361 | |
Average | 0.537 | 9.016 | 1.613 | 0.574 | 9.359 | 1.416 |
When compared with neuroradiologist’s contours, clinician contours had better Dice co-efficient and lower average Hausdorff distance while elements contours had lower Hausdorff maximal distances although none of these differences were found to be significant (Tables 3,4, Figures 3,4).
Table 3
Left hippocampus | Right hippocampus | ||||||
---|---|---|---|---|---|---|---|
Dice co-efficient | Hausdorff | Dice co-efficient | Hausdorff | ||||
Max (mm) | Mean (mm) | Max (mm) | Mean (mm) | ||||
Radiologist vs. RO1 | 0.634 | 8.172 | 1.217 | 0.647 | 7.432 | 1.121 | |
Radiologist vs. RO2 | 0.667 | 8.620 | 1.161 | 0.698 | 8.056 | 1.009 | |
Radiologist vs. RO3 | 0.664 | 7.913 | 1.103 | 0.668 | 7.169 | 1.045 | |
Average | 0.655 | 8.235 | 1.160 | 0.671 | 7.552 | 1.058 |
Table 4
Left hippocampus | Right hippocampus | ||||||
---|---|---|---|---|---|---|---|
Dice co-efficient | Hausdorff | Dice co-efficient | Hausdorff | ||||
Max (mm) | Mean (mm) | Max (mm) | Mean (mm) | ||||
Radiologist vs. elements | 0.598 | 7.078 | 1.268 | 0.632 | 6.026 | 1.134 |
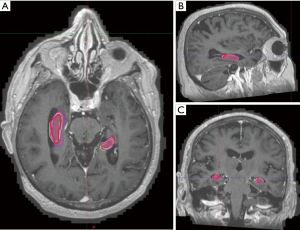
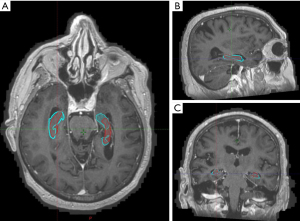
Discussion
Since the publication of RTOG 0933, the potential for hippocampal-avoidance in neurocognitive preservation for whole-brain treatment was established (2). This potential was of great importance as neurocognitive toxicity has been a major contributor in the diminishing use of traditional WBRT for the treatment of multiple brain metastases. The Phase III NRG CC001 randomised trial results now have confirmed HA-WBRT with memantine to be superior to WBRT in diffuse cognitive parameters as well as quality of life with no difference in overall or intracranial progression free survival (3). However, workflow for HA-WBRT is significantly more complicated, requiring recent MRI brain acquisition and fusion, OAR contouring, and complex planning.
Contouring of the hippocampi can be challenging and a RTOG contouring atlas was published to aid in their delineation (13). The first portion of this study assessed the conformality of clinician hippocampal contours using the RTOG atlas. An average Dice co-efficient of 0.766 and average Hausdorff distance of less than 1mm confirmed that reasonable conformality can be achieve using this atlas. On visual inspection of the manual clinician contours, the main point of difference was the superior or inferior slice of the contours with no significant differences at the body of the hippocampi. This compares favourably to the central review of OARs on NRG CC001, where only 65.5% of contours were per protocol (3).
With reasonable conformality confirmed between clinician contours, we then compared these to the atlas-based auto-contours generated by Elements TPS, a MRI-atlas based system that is commonly used in radiation oncology departments that deliver Linac-based cranial radiosurgery treatment. The comparison saw a much lower Dice co-efficient and almost doubling of the Hausdorff maximal distances to 9.0 mm for the left and 9.4 mm for the right hippocampus, denoting poor conformality between manual and auto-contours. There have been limited studies assessing manual versus auto-contouring of the hippocampi. One recent study utilized NeuroQuant software, a fully-automated software originally designed for the quantification of brain atrophy in Alzheimer Disease, to contour the hippocampi of 100 patients. These contours were reviewed by a sole radiation oncologist with no manual intervention required in 99 of these cases (14), suggesting that this software may perform better than Elements for hippocampi contouring. In addition, deep-learning auto-contouring has shown promise in other anatomical areas, especially in the head and neck region (15-22). Further improvements in computing power and neural network training may see its more widespread use in the future.
A possible reason for the differences in contours may be partly explained by the third comparison, in which the clinician and MRI-atlas software contours were compared with those done by a neuroradiologist on the same 10 patient datasets. The neuroradiologist had no prior appraisal of the RTOG atlas and contours were anatomy based using the MRI sequences. Although there were numerical improvements of the dice co-efficients and average Hausdorff measurements between radiation oncologist contours with the neuroradiologist in comparison to elements with radiologist contours, there were no statistical differences in any of the conformality indices. This may indicate that the Elements atlas was built using anatomical contours which differ from the RTOG atlas.
Conclusions
The current results show reasonable conformality of clinician manual contouring using the RTOG Atlas and validate its use in the clinical setting. Differences in clinician contours when compared with neuroradiologist contours may be due to differences in RTOG-atlas guidelines adhered to by clinicians compared to a more anatomy-based contouring by the neuroradiologist. Whilst elements TPS could be utilized as a starting point, contours currently will require modification by clinicians to conform to the RTOG atlas. Future study into other auto-contouring methods and systems and contouring by radiation therapists after training will be undertaken.
Acknowledgments
This study was conducted at the Chris O’Brien Lifehouse, Camperdown, Australia.
Funding: None.
Footnote
Reporting Checklist: The authors have completed the STROBE reporting checklist. Available at https://dx.doi.org/10.21037/tro-21-15
Conflicts of Interest: All authors have completed the ICMJE uniform disclosure form (available at https://dx.doi.org/10.21037/tro-21-15). The authors have no conflicts of interest to declare.
Ethical Statement: The authors are accountable for all aspects of the work in ensuring that questions related to the accuracy or integrity of any part of the work are appropriately investigated and resolved. The study was conducted in accordance with the Declaration of Helsinki (as revised in 2013). The study was approved by the Sydney Local Health District – Royal Prince Alfred Ethics Review Committee (reference: X20-0304 & 2020/ETH01446). Informed consent was not required as no patient information was attached to the MRI nor was any patient contact or information otherwise required for the purposes of this study.
Open Access Statement: This is an Open Access article distributed in accordance with the Creative Commons Attribution-NonCommercial-NoDerivs 4.0 International License (CC BY-NC-ND 4.0), which permits the non-commercial replication and distribution of the article with the strict proviso that no changes or edits are made and the original work is properly cited (including links to both the formal publication through the relevant DOI and the license). See: https://creativecommons.org/licenses/by-nc-nd/4.0/.
References
- Brown PD, Ahluwalia MS, Khan OH, et al. Whole-Brain Radiotherapy for Brain Metastases: Evolution or Revolution? J Clin Oncol 2018;36:483-91. [Crossref] [PubMed]
- Gondi V, Pugh SL, Tome WA, et al. Preservation of memory with conformal avoidance of the hippocampal neural stem-cell compartment during whole-brain radiotherapy for brain metastases (RTOG 0933): a phase II multi-institutional trial. J Clin Oncol 2014;32:3810-6. [Crossref] [PubMed]
- Brown PD, Gondi V, Pugh S, et al. Hippocampal Avoidance During Whole-Brain Radiotherapy Plus Memantine for Patients With Brain Metastases: Phase III Trial NRG Oncology CC001. J Clin Oncol 2020;38:1019-29. [Crossref] [PubMed]
- Sharp G, Fritscher KD, Pekar V, et al. Vision 20/20: perspectives on automated image segmentation for radiotherapy. Med Phys 2014;41:050902. [Crossref] [PubMed]
- Rohlfing T, Brandt R, Menzel R, et al. Quo Vadis, Atlas-Based Segmentation? The Handbook of Medical Image Analysis: Segmentation and Registration Models, Kluwer 2007. doi:
10.1007/0-306-48608-3 . - La Macchia M, Fellin F, Amichetti M, et al. Systematic evaluation of three different commercial software solutions for automatic segmentation for adaptive therapy in head-and-neck, prostate and pleural cancer. Radiat Oncol 2012;7:160. [Crossref] [PubMed]
- Zabel WJ, Conway JL, Gladwish A, et al. Clinical Evaluation of Deep Learning and Atlas-Based Auto-Contouring of Bladder and Rectum for Prostate Radiation Therapy. Pract Radiat Oncol 2021;11:e80-9. [Crossref] [PubMed]
- Wong WK, Leung LH, Kwong DL. Evaluation and optimization of the parameters used in multiple-atlas-based segmentation of prostate cancers in radiation therapy. Br J Radiol 2016;89:20140732. [Crossref] [PubMed]
- Van de Velde J, Wouters J, Vercauteren T, et al. Optimal number of atlases and label fusion for automatic multi-atlas-based brachial plexus contouring in radiotherapy treatment planning. Radiat Oncol 2016;11:1. [Crossref] [PubMed]
- Larrue A, Gujral D, Nutting C, et al. The impact of the number of atlases on the performance of automatic multi-atlas contouring. Phys Med 2015;31:e30. [Crossref]
- Yeo UJ, Supple JR, Taylor ML, et al. Performance of 12 DIR algorithms in low-contrast regions for mass and density conserving deformation. Med Phys 2013;40:101701. [Crossref] [PubMed]
- Zhong H, Kim J, Chetty IJ. Analysis of deformable image registration accuracy using computational modeling. Med Phys 2010;37:970-9. [Crossref] [PubMed]
- Gondi V, Tome WA, Rowley H, et al. Hippocampal contouring: a contouring atlas for RTOG 0933. Available online: http://www.rtog.org/CoreLab/ContouringAtlases/HippocampalSparing.aspx
- Feng CH, Cornell M, Moore KL, et al. Automated contouring and planning pipeline for hippocampal-avoidant whole-brain radiotherapy. Radiat Oncol 2020;15:251. [Crossref] [PubMed]
- LeCun Y, Bengio Y, Hinton G. Deep learning. Nature 2015;521:436-44. [Crossref] [PubMed]
- van Dijk LV, Van den Bosch L, Aljabar P, et al. Improving automatic delineation for head and neck organs at risk by Deep Learning Contouring. Radiother Oncol 2020;142:115-23. [Crossref] [PubMed]
- Meyer P, Noblet V, Mazzara C, et al. Survey on deep learning for radiotherapy. Comput Biol Med 2018;98:126-46. [Crossref] [PubMed]
- Ibragimov B, Xing L. Segmentation of organs-at-risks in head and neck CT images using convolutional neural networks. Med Phys 2017;44:547-57. [Crossref] [PubMed]
- Liang S, Tang F, Huang X, et al. Deep-learning-based detection and segmentation of organs at risk in nasopharyngeal carcinoma computed tomographic images for radiotherapy planning. Eur Radiol 2019;29:1961-7. [Crossref] [PubMed]
- Lustberg T, van Soest J, Gooding M, et al. Clinical evaluation of atlas and deep learning based automatic contouring for lung cancer. Radiother Oncol 2018;126:312-7. [Crossref] [PubMed]
- Men K, Dai J, Li Y. Automatic segmentation of the clinical target volume and organs at risk in the planning CT for rectal cancer using deep dilated convolutional neural networks. Med Phys 2017;44:6377-89. [Crossref] [PubMed]
- Ibragimov B, Toesca D, Chang D, et al. Combining deep learning with anatomical analysis for segmentation of the portal vein for liver SBRT planning. Phys Med Biol 2017;62:8943-58. [Crossref] [PubMed]
Cite this article as: Lo H, Estoesta P, Mackonis EC, Tse R, Harris G, Van Camp L, Choong ES. Clinical evaluation of RTOG-atlas based contouring, MRI-atlas auto-contouring, and neuroradiologist contouring of the hippocampi for hippocampal avoidance whole-brain radiotherapy. Ther Radiol Oncol 2021;5:19.