Using computed tomography-based radiomics to predict outcomes for hepatocellular carcinoma patients receiving stereotactic body radiotherapy
Introduction
In recent decades, stereotactic body radiotherapy (SBRT) has emerged as an attractive option for tumor ablation, including in hepatocellular carcinoma (HCC) (1,2). However, SBRT is still usually reserved for salvage therapy in patients who are unsuitable or refractory to other locoregional therapies, probably due to the lack of randomized controlled trials and reliable predictors for evaluating therapeutic efficacy.
Radiomics is a burgeoning research field that focuses on the extraction of large-scale image data and their analysis via high-throughput mining of quantitative imaging features (3-6). The field has gradually gained importance in oncology for its value in diagnostic, prognostic, and predictive accuracy (7). Using medical imaging data for diagnosis and prognostication stems from the notion that tumors of a specific genotype display heterogeneous phenotypes and anatomic variations (8). The application of radiomics involves the use of personalized image data that can be incorporated within the clinical decision-support system, enabling the construction of models that predict therapeutic outcomes (9). As sophisticated tools for image analysis generate large amounts of data, machine learning (ML) has emerged as a powerful methodology for constructing precise predictive models, thus improving predictive performance (10).
In HCC, radiomics is particularly valuable because this cancer is most often diagnosed via dynamic three-phase computed tomography (CT) scans, thus potentiating the use of image features to predict therapeutic responses. To the best of our knowledge, there have been no studies using CT-based radiomics to predict the effect of SBRT on HCC. Therefore, we aimed to propose a radiomics-based ML strategy for the prediction of local response (LR) after SBRT. We present the following article in accordance with the PRISMA reporting checklist (available at https://dx.doi.org/10.21037/tro-21-8).
Methods
Patients for clinical evaluation and radiomic analysis
Clinical data of patients with HCC who received SBRT at our institution between 2007 and 2016 were retrospectively reviewed. For radiomic analysis, patients who underwent locoregional ablative treatments in the past were excluded. Enrolled patients had undergone dynamic CT before radiotherapy and follow-up CT to evaluate responses. Follow-up time was obtained from the date of treatment to the last outpatient visit, with a median follow-up time of 26.6 months. Because of the retrospective nature of this study, we obtained approval from our Institutional Review Board for a waiver of informed consent (IRB number: 1-107-05-016, analysis of treatment outcome of patients with hepatic tumors). This study was conducted in accordance with the Declaration of Helsinki (as revised in 2013).
SBRT and clinical endpoints
All patients were treated with SBRT using the Cyberknife image-guided radiosurgery system (Accuray, Sunnyvale, CA) as described previously (11-13). The modified Response Evaluation Criteria in Solid Tumors (mRECIST) system was used to assess therapeutic responses (14). Tumors achieving complete and partial responses, as per mRECIST criteria, were considered as having local control (LC). The LC that occurred during the first year after treatment was considered as a 1-year LC or within 1-year response (W1R) in our study. End response was defined as a complete/partial response achieved by the end of the follow-up period (EndR). Tumors within the radiotherapy field that did not show increase in size at the end of the follow-up period were considered to have attained in-field failure-free (IFFF). EndR and IFFF were used to evaluate two different aspects of clinical response, with the former aiming to identify the final responders and the latter to address progressive events.
Extraction of radiomic features
Complete dynamic, multiphasic CT scans with contrast were retrieved individually in the Digital Imaging and Communications in Medicine (DICOM) format. All scans were performed with a tube voltage of 120 kVp and a pitch of 0.984. The images were then imported to the Computational Environment for Radiotherapy Research (CERR) platform based on MATLAB (MathWorks, Naticks, MA, 2017b) for radiation planning analysis. For each contrast-phase CT scan [arterial (A), portovenous (E), and delayed (D)], tumor regions were contoured by two experienced radiation oncologists (WYH and CHL) and modified by another experienced radiologist (WCC) to minimize delineation uncertainty (Figure 1). Each slice was resized to 128×128 voxels using bicubic interpolation (0.8301×0.8301×3 mm3 for each voxel). The intensity distribution was standardized by histogram equalization. To reduce image noise and increase the sensitivity of radiomic analyses, a bin width of 25 Hounsfield units was used for discretization prior to global texture analysis (15). Radiomic features were subsequently extracted and divided into 4 categories: first-order statistics, shape, global, and texture. For matrix-based texture features, different combinations of quantization [equal probability (Ep) and uniform quantization (U)] of gray levels (8, 16, 32, and 64) were performed (16). Incorporating CT scans from each phase, the training datasets combined features from different CT phases, quantization methods, and gray levels, and were termed AEp (8, 16, 32, and 64), AU (8, 16, 32, and 64), EEp (8, 16, 32, and 64), EU (8, 16, 32, and 64), DEp (8, 16, 32, and 64), and DU (8, 16, 32, and 64). Altogether, there were 41 tumors identified and 46 radiomic features prior further processing.
Machine learning characterization
Data augmentation and adjustment of imbalanced data
Oversampling with bootstrapping was used to expand our data population to over 5,000 samples. Synthetic Minority Oversampling Technique (SMOTE) was then used to adjust the imbalanced data. For the minority class, SMOTE is advantageous for making the decision region more general and improving the classifier performance (17).
All 41 identified tumors were used for radiomic analyses. 78% (32/41) of the initial samples were extracted randomly and oversampled for training. The remaining 22% (9/41) was oversampled in a similar way for subsequent testing. The oversampled data set consisted of 6,502 samples, with 5,201 training (80%) and 1,301 testing (20%) samples. Both of the training and testing cohorts were then balanced by SMOTE for positive and negative LRs.
Feature selection
The radiomic features extracted from the contoured region of each tumor were averaged and subsequently normalized across the cohort. To select the radiomic features, a regression method used to improve prediction accuracy by incorporating penalized estimation functions known as Least Absolute Shrinkage and Selection Operator (LASSO) was used (18). LASSO started feature selection by tuning a parameter (λ). During this process, most covariate coefficients were shrunk to zero, and the remaining features with non-zero coefficients were selected. For each training set, which included the training features with the corresponding training responses (i.e., W1R, ENdR, and IFFF), the features selected by LASSO were used to build the classifiers.
Support vector machine (SVM) and logistic regression (LRG) classifiers
With high prediction accuracy in various clinical settings (19,20), SVM and LRG were adopted in our study to construct classifiers for LR. The SVM classifier deals with non-linear interaction and was used to discriminate whether a LR was achieved or not (21). The penalty parameter C was set to 1 to determine the tradeoff between fitting error and model complexity. Radial basis function was used as the kernel function in our SVM classifier. The LRG was used to predict the likelihood of positive LR, and a probability equal to 0.5 was set as the minimum threshold to determine the predicted class.
Evaluation of model performance
The model performance of SVM and LRG classifiers were evaluated for accuracy, sensitivity, and specificity after 10-fold cross-validation in the training cohorts. F1 score was used to evaluate the model robustness in the testing cohorts. The classifier with the highest mean F1 scores for the three LRs was chosen as the candidate model. Receiver operating characteristic (ROC) curves were then used to assess the output quality with area under curve (AUC) and 5-fold cross-validation. All the functions used in our analyses were based on Python.
Statistical analysis
For the evaluation of radiomic data processing methods, the 50th percentile of accuracy was used as the threshold. The numbers of processing methods with an accuracy above the 50th percentile were identified and used for comparison of parameter robustness. Kaplan-Meier (KM) analysis with log-rank test was used to evaluate the effect of therapeutic response on survival. P value <0.05 was considered significant. All statistical analyses and survival calculation were performed in R.
Results
In-patient demographics
Overall, 172 patients receiving SBRT for HCC were retrospectively reviewed in our institution. Patient information and therapeutic outcomes are summarized in Table 1. The median tumor size was 5.4 cm, ranging from 0.8 cm to as large as 20.1 cm. There were 76.7% CP class A patients and 23.3% CP class B patients in the overall cohort. In this cohort, 36.6% patients presented with portal vein thrombosis (PVT). Patients in the radiomic cohort showed similar characteristics with those in the overall cohort. The median tumor size was 5 cm, with the largest treated tumor being 13 cm. There were 84.8% CP class A patients and 15.2% CP class B patients. Out of the 33 patients, 21.2% showed PVT. The radiation dose ranges were also similar between the two cohorts (25–65 Gy in 4–6 fractions in the overall cohort; 32–60 Gy in 4–6 fractions in the radiomic cohort).
Table 1
Baseline features | Overall cohort | Radiomic cohort |
---|---|---|
Patient number | 172 | 33 |
Tumor size (median) | 5.4 cm (0.8–20.1 cm) | 5 cm (1.8–13 cm) |
Age (SD) | 63±13 | 68.5±12 |
Sex | Male (76.7%), Female (23.3%) | Male (63.6%), Female (36.4%) |
CP class | A (76.7%), B (23.3%) | A (84.8%), B (15.2%) |
ECOG | 0 (47.7%), 1 (38.4%), 2 (13.9%) | 0 (42.4%), 1 (39.3%), 2 (18.3%) |
PVT | 36.6% | 21.2% |
Dose | 25–65 Gy/4–6 fx | 32–60 Gy/4–6 fx |
Median F/u (month) | 32.8 | 26.6 |
SD, standard deviation; CP class, Child-Pugh class; ECOG, Eastern Cooperative Oncology Group; PVT, portal vein thrombosis; Gy, Gray; fx, fractions; F/u, follow-up.
Construction and evaluation of the predictive models
Accounting for the three types of LR, a total of 72 datasets were analyzed. Using SVM, classifiers with the highest accuracy (98.7%) were built from features processed in A phase with Ep quantization and 32 gray levels (AEp32) for W1R (Figure 2A). For EndR, features processed in E phase with Ep quantization and 8 gray levels (EEp8) had the highest accuracy [99.3%, 95% confidence interval (CI): 91.2–99.5%]; and for IFFFR, features processed in A phase with Ep quantization and 16 gray levels (AEp16) corresponded to the highest accuracy (99.7%, 95% CI: 99.5–99.8%). When we looked at sensitivity and specificity, CT scans in the A and D phases generally presented with higher sensitivity and specificity than in the E phase (Figure S1A,S1B). Interestingly, AEp8 exhibited the highest sensitivity (98.2%, 95% CI: 97.9–99.0%) for W1R and the highest specificity (76.5%, 95% CI: 72.1–77.3%) for EndR. However, the specificity was generally low for IFFF in all datasets.
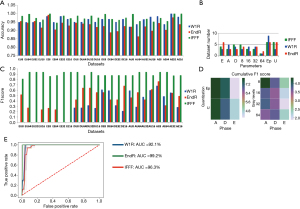
When examining the processing sources and methods, we identified roughly similar distribution of three phases for the three LRs in the 50th percentile of accuracy (Figure 2B). Moreover, classifiers with higher accuracy were mostly constructed by features with 8, 16, or 32 gray levels, not 64 gray levels. For the quantization methods, features processed with Ep quantization corresponded to higher accuracy for W1R (n=9), compared with those processed with U quantization (n=3). In the testing cohort, dataset processed in the A phase with Ep quantization and 8 gray levels (AEp8) exhibited the highest mean F1 scores for the three LRs (0.7995) (Figure 2C). In separate conditions, Ep quantization and 8 gray levels in the A phase had the highest cumulative F1 scores compared to other parameters (Figure 2D). Based on the above findings, AEp8 was chosen as the candidate model. ROC curve was plotted, and we observed the AUC for IFFF was the highest (AUC =99.2%, 95% CI: 99.0–93.2%), followed by EndR (AUC =96.3%, 95% CI: 96.0–96.9%) and W1R in AEp8 (AUC =92.1%, 95% CI: 91.8–93.4%) (Figure 2E). For AEp8, the optimal λ for LASSO was 0.00045 and the most potential feature for W1R was Long Run High Gray-Level Emphasis (LRHGE, coefficient =−5.135). For EndR and IFFF, Long Zone High Gray-Level Emphasis (LZLGE, λ =0.00039, coefficient =7.207) and Long Run Low Gray-Level Emphasis (LRLGE, λ =0.00035, coefficient =−3.587) were the most predictive features, respectively.
For LRG, the classifiers with the highest accuracy for W1R, EndR, and IFFF were built from DU32 (98.2%, 95% CI: 97.6–98.5%), DEp64 (98.1%, 95% CI: 97.6–99.0%) and EU64 (99.5%, 95% CI: 99.1–99.7%) (Figure 3A). We found no datasets presented with both high sensitivity and specificity for the three LRs (Figure S1C,S1D). Like SVM, more E phases were noted in the 50th percentile of accuracy for EndR (n=7); and the distribution of accuracy for Ep and U quantization methods demonstrated greater discrepancy, as compared to SVM (Figure 3B). In the testing cohort, AEp64 was found to outperform other datasets in terms of LR prediction (mean F1 score for the three LRs =0.7783), even though the Ep quantization or 8 gray levels had higher cumulative F1 scores (Figure 3C,D). The ROC curve for AEp64 revealed that IFFF had the highest AUC (99.2%, 95% CI: 98.7–99.6%), whereas EndR exhibited the lowest AUC (86.1%, 95% CI: 85.4–88.2%) (Figure 3E). In AEp64, the most predictive feature were Gray-Level Non-Uniformity (GLN, λ =0.00046, coefficient =12.566), Long Gray-Level Run Emphasis (LGRE, λ =0.000395, coefficient =7.019), and LRHGE (λ =0.00036, coefficient =−7.294) for W1R, EndR, and IFFF, respectively.
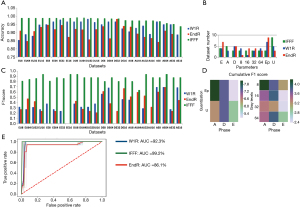
Association of W1R with 1-year OS
Patients with W1R had significantly higher survival (P<0.001) (Figure 4). After excluding patients with missing data for survival evaluation, the remaining cohort comprised of 156 samples. The patients with W1R had a median survival of 27.4 months, compared to 8 months in patients without W1R. This result suggested that achieving W1R was associated with better survival probability.
Discussion
Despite great therapeutic outcomes and advances in radiation techniques, the treatment of HCC using SBRT remains challenging. The benefit of SBRT is stronger for CP class A patients, who can receive higher radiation doses and more aggressive fractionation schedules with lower toxicity (1,22-26), than for CP class B patients (23,27). Moreover, several studies also reported a clear survival benefit in CP class A compared to CP class B (23,25,27,28). Therefore, the balance of benefits and risks should be carefully evaluated. In our study, patients undergoing SBRT were mostly in the CP class A (76.7%, Table 1). However, there were still 23.3% CP class B patients. The latter had poorer liver function and thus the prescription of a higher dose was limited. Additionally, as high as 40.7% patients with W1R had PVT, which further reduced the chance of survival benefit (29,30). Nonetheless, SBRT is still considered to be of therapeutic benefit for patients with PVT (31). In the evaluable 53 cases of PVT, EndR was achieved in 42 cases (79.2%), which represented a higher percentage than in patients who received Sorafenib alone (7% based on mRECIST) in a comparable study (32). After a median follow-up of 32.8 months, the 1-year LC in the overall cohort was 85.4%. This relatively lower LC could be explained by various definitions of LC across studies (1,22-26). In our analysis, only those having at least a partial response were considered as achieving LC. In a similar cohort in Taiwan, the reported 1-year in-field control was 85.3% (33). Even though this finding was quite similar to our result, their LC was defined as the absence of new lesion or increase in tumor size, which was less strict compared to our definition. Therefore, our result might be better and still comparable to the literature.
Scorsetti et al. used a similar definition of LC to that in our study and found that higher LC contributed to higher OS in HCC patients after SBRT (28). In support of this, patients with W1R were shown in our study to have significantly longer survival than those without W1R (Figure 3). Therefore, achieving LC seems to be of great clinical significance. In several previous reports, LC appears to be determined by tumor size (28,34). However, as aggressive tumors tend to exhibit increased intratumoral heterogeneity, the sole use of tumor size in predicting LR appears insufficient and inappropriate (35-37). In addition to histologic and genomic study, radiomics is another non-invasive approach that enables a spatiotemporal and quantitative measurement of tumoral heterogeneity (38). In the current study, 26 out of 46 features were based on the matrix manipulation, which were related to the quantitative description of size and intensity variations of the connected sub-regions. The most predictive features selected by LASSO for the three LRs were LRHGE, LZLGE, and LRLGE, respectively. These metrics quantify the heterogeneity in size and intensity within tumor volumes in CT images, which was in agreement with a previous study describing the significance of Gray-Level Uniformity for prognosis in HCC patients receiving radiotherapy (39). These features are related to the spatial correlation with emphasis on gray levels, possibly capturing the intratumoral heterogeneity. However, the value of these features is attributed to the type of CT scans and the preprocessing methods. In our study, the features extracted from the A phase had higher mean F1 scores in either SVC or LRG classifiers. This finding is in line with the fact that the development of HCC tumor frequently involves neovascularization of unpaired arteries without associated portal tracts (40,41) and consequently that contrast enhancement in the A phase with early wash-out in the E phase is a widely accepted diagnostic criteria. Therefore, features in the A phase are believed to be much more informative, potentiating the prediction for LR. Unlike in other studies (42,43), we used two quantization methods and identified that Ep quantization with 8 and 64 gray levels performed better on the A phase CT scans. Ep quantization method attempts to define a decision threshold in the tumor volume while maintaining the same number of voxels after quantization (16). Ep quantization and 8 gray levels showed higher cumulative F1 scores individually in the A phase in the SVM and LRG classifiers (Figure 2D). The SVM classifier based on AEp8 also exhibited the highest mean F1 score for the three LRs, suggesting the feasibility of these parameters for LR prediction.
Even though we hereby proposed a radiomics-based ML strategy for SBRT in HCC, some limitations of current predictive models still need to be addressed prior to clinical application. First, the sample size was small in the initial dataset. Although we used an oversampling technique, the augmented data retained the intrinsic characteristics of the small number of original tumors, restricting its general utility. An alternative is to use image augmentation, generating large number of tumor images for training. However, this strategy also suffers from the similar limitation. Second, the tumors were not segmented automatically, thus the uncertainty of the peripheral regions might be increased. Future development of tumor segmentation out of normal liver could help refine this procedure. Furthermore, we did not adopt image filtering such as Laplacian or Gaussian filters, which could have enhanced the reproducibility of feature extraction. Finally, since low specificity was consistently observed for SVM and LRG, IFFF seemed poorly defined and required further elucidation. Once more patients are included with clearly defined target response, we believe that our model will exhibit some improved performance in the future.
Conclusions
In conclusion, this is the first study to propose a radiomics-based predictive model for SBRT efficacy in patients with HCC. The findings warrant further studies in larger populations to confirm the feasibility of using our radiomics-based model in the clinic.
Acknowledgments
The authors thank the Cancer Registry Group of Tri-Service General Hospital for the clinical data support.
Funding: None.
Footnote
Reporting Checklist: The authors have completed the PRISMA reporting checklist. Available at https://dx.doi.org/10.21037/tro-21-8
Conflicts of Interest: All authors have completed the ICMJE uniform disclosure form (available at https://dx.doi.org/10.21037/tro-21-8). Hsing-Lung Chao and Jing-Min Hwang serve as unpaid editorial board members of Therapeutic Radiology and Oncology from May 2020 to Apr 2022. The other authors have no conflicts of interest to declare.
Ethical Statement: The authors are accountable for all aspects of the work in ensuring that questions related to the accuracy or integrity of any part of the work are appropriately investigated and resolved. The study was conducted in accordance with the Declaration of Helsinki (as revised in 2013). The study was approved by Institutional Review Board (NO.: 1-107-05-016) and individual consent for this retrospective analysis was waived.
Open Access Statement: This is an Open Access article distributed in accordance with the Creative Commons Attribution-NonCommercial-NoDerivs 4.0 International License (CC BY-NC-ND 4.0), which permits the non-commercial replication and distribution of the article with the strict proviso that no changes or edits are made and the original work is properly cited (including links to both the formal publication through the relevant DOI and the license). See: https://creativecommons.org/licenses/by-nc-nd/4.0/.
References
- Bujold A, Massey CA, Kim JJ, et al. Sequential phase I and II trials of stereotactic body radiotherapy for locally advanced hepatocellular carcinoma. J Clin Oncol 2013;31:1631-9. [Crossref] [PubMed]
- Wahl DR, Stenmark MH, Tao Y, et al. Outcomes After Stereotactic Body Radiotherapy or Radiofrequency Ablation for Hepatocellular Carcinoma. J Clin Oncol 2016;34:452-9. [Crossref] [PubMed]
- El Naqa I, Grigsby P, Apte A, et al. Exploring feature-based approaches in PET images for predicting cancer treatment outcomes. Pattern Recognit 2009;42:1162-71. [Crossref] [PubMed]
- Gillies RJ, Anderson AR, Gatenby RA, et al. The biology underlying molecular imaging in oncology: from genome to anatome and back again. Clin Radiol 2010;65:517-21. [Crossref] [PubMed]
- Kumar V, Gu Y, Basu S, et al. Radiomics: the process and the challenges. Magn Reson Imaging 2012;30:1234-48. [Crossref] [PubMed]
- Lambin P, Rios-Velazquez E, Leijenaar R, et al. Radiomics: extracting more information from medical images using advanced feature analysis. Eur J Cancer 2012;48:441-6. [Crossref] [PubMed]
- Lambin P, Leijenaar RTH, Deist TM, et al. Radiomics: the bridge between medical imaging and personalized medicine. Nat Rev Clin Oncol 2017;14:749-62. [Crossref] [PubMed]
- Aerts HJ, Velazquez ER, Leijenaar RT, et al. Decoding tumour phenotype by noninvasive imaging using a quantitative radiomics approach. Nat Commun 2014;5:4006. [Crossref] [PubMed]
- Lambin P, van Stiphout RG, Starmans MH, et al. Predicting outcomes in radiation oncology--multifactorial decision support systems. Nat Rev Clin Oncol 2013;10:27-40. [Crossref] [PubMed]
- Parmar C, Grossmann P, Bussink J, et al. Machine Learning methods for Quantitative Radiomic Biomarkers. Sci Rep 2015;5:13087. [Crossref] [PubMed]
- Huang WY, Jen YM, Lee MS, et al. Stereotactic body radiation therapy in recurrent hepatocellular carcinoma. Int J Radiat Oncol Biol Phys 2012;84:355-61. [Crossref] [PubMed]
- Lo CH, Liu MY, Lee MS, et al. Comparison Between Child-Turcotte-Pugh and Albumin-Bilirubin Scores in Assessing the Prognosis of Hepatocellular Carcinoma After Stereotactic Ablative Radiation Therapy. Int J Radiat Oncol Biol Phys 2017;99:145-52. [Crossref] [PubMed]
- Shen PC, Chang WC, Lo CH, et al. Comparison of Stereotactic Body Radiation Therapy and Transarterial Chemoembolization for Unresectable Medium-Sized Hepatocellular Carcinoma. Int J Radiat Oncol Biol Phys 2019;105:307-18. [Crossref] [PubMed]
- Llovet JM, Di Bisceglie AM, Bruix J, et al. Design and endpoints of clinical trials in hepatocellular carcinoma. J Natl Cancer Inst 2008;100:698-711. [Crossref] [PubMed]
- Huynh E, Coroller TP, Narayan V, et al. CT-based radiomic analysis of stereotactic body radiation therapy patients with lung cancer. Radiother Oncol 2016;120:258-66. [Crossref] [PubMed]
- Vallières M, Freeman CR, Skamene SR, et al. A radiomics model from joint FDG-PET and MRI texture features for the prediction of lung metastases in soft-tissue sarcomas of the extremities. Phys Med Biol 2015;60:5471-96. [Crossref] [PubMed]
- Chawla NV, Bowyer KW, Hall LO, et al. SMOTE: Synthetic Minority Over-sampling Technique. J Artif Intell Res 2002;16:321-57. [Crossref]
- Tibshirani R. Regression shrinkage and selection via the lasso: a retrospective. J R Statist Soc B 2011;73:273-82. [Crossref]
- Yahya N, Ebert MA, Bulsara M, et al. Statistical-learning strategies generate only modestly performing predictive models for urinary symptoms following external beam radiotherapy of the prostate: A comparison of conventional and machine-learning methods. Med Phys 2016;43:2040. [Crossref] [PubMed]
- Lee HC, Yoon HK, Nam K, et al. Derivation and Validation of Machine Learning Approaches to Predict Acute Kidney Injury after Cardiac Surgery. J Clin Med 2018;7:322. [Crossref] [PubMed]
- Gultepe E, Green JP, Nguyen H, et al. From vital signs to clinical outcomes for patients with sepsis: a machine learning basis for a clinical decision support system. J Am Med Inform Assoc 2014;21:315-25. [Crossref] [PubMed]
- Sanuki N, Takeda A, Oku Y, et al. Stereotactic body radiotherapy for small hepatocellular carcinoma: a retrospective outcome analysis in 185 patients. Acta Oncol 2014;53:399-404. [Crossref] [PubMed]
- Lasley FD, Mannina EM, Johnson CS, et al. Treatment variables related to liver toxicity in patients with hepatocellular carcinoma, Child-Pugh class A and B enrolled in a phase 1-2 trial of stereotactic body radiation therapy. Pract Radiat Oncol 2015;5:e443-9. [Crossref] [PubMed]
- Su TS, Liang P, Lu HZ, et al. Stereotactic body radiation therapy for small primary or recurrent hepatocellular carcinoma in 132 Chinese patients. J Surg Oncol 2016;113:181-7. [Crossref] [PubMed]
- Jeong Y, Jung J, Cho B, et al. Stereotactic body radiation therapy using a respiratory-gated volumetric-modulated arc therapy technique for small hepatocellular carcinoma. BMC Cancer 2018;18:416. [Crossref] [PubMed]
- Nabavizadeh N, Waller JG, Fain R 3rd, et al. Safety and Efficacy of Accelerated Hypofractionation and Stereotactic Body Radiation Therapy for Hepatocellular Carcinoma Patients With Varying Degrees of Hepatic Impairment. Int J Radiat Oncol Biol Phys 2018;100:577-85. [Crossref] [PubMed]
- Cárdenes HR, Price TR, Perkins SM, et al. Phase I feasibility trial of stereotactic body radiation therapy for primary hepatocellular carcinoma. Clin Transl Oncol 2010;12:218-25. [Crossref] [PubMed]
- Scorsetti M, Comito T, Cozzi L, et al. The challenge of inoperable hepatocellular carcinoma (HCC): results of a single-institutional experience on stereotactic body radiation therapy (SBRT). J Cancer Res Clin Oncol 2015;141:1301-9. [Crossref] [PubMed]
- Schöniger-Hekele M, Müller C, Kutilek M, et al. Hepatocellular carcinoma in Central Europe: prognostic features and survival. Gut 2001;48:103-9. [Crossref] [PubMed]
- Yeh SA, Chen YS, Perng DS. The role of radiotherapy in the treatment of hepatocellular carcinoma with portal vein tumor thrombus. J Radiat Res 2015;56:325-31. [Crossref] [PubMed]
- Shui Y, Yu W, Ren X, et al. Stereotactic body radiotherapy based treatment for hepatocellular carcinoma with extensive portal vein tumor thrombosis. Radiat Oncol 2018;13:188. [Crossref] [PubMed]
- Gavanier M, Ayav A, Sellal C, et al. CT imaging findings in patients with advanced hepatocellular carcinoma treated with sorafenib: Alternative response criteria (Choi, European Association for the Study of the Liver, and modified Response Evaluation Criteria in Solid Tumor (mRECIST)) versus RECIST 1.1. Eur J Radiol 2016;85:103-12. [Crossref] [PubMed]
- Que J, Kuo HT, Lin LC, et al. Clinical outcomes and prognostic factors of cyberknife stereotactic body radiation therapy for unresectable hepatocellular carcinoma. BMC Cancer 2016;16:451. [Crossref] [PubMed]
- Kang JK, Kim MS, Cho CK, et al. Stereotactic body radiation therapy for inoperable hepatocellular carcinoma as a local salvage treatment after incomplete transarterial chemoembolization. Cancer 2012;118:5424-31. [Crossref] [PubMed]
- Fidler IJ. Critical factors in the biology of human cancer metastasis: twenty-eighth G.H.A. Clowes memorial award lecture. Cancer Res 1990;50:6130-8. [PubMed]
- Yokota J. Tumor progression and metastasis. Carcinogenesis 2000;21:497-503. [Crossref] [PubMed]
- Campbell PJ, Yachida S, Mudie LJ, et al. The patterns and dynamics of genomic instability in metastatic pancreatic cancer. Nature 2010;467:1109-13. [Crossref] [PubMed]
- Sanduleanu S, Woodruff HC, de Jong EEC, et al. Tracking tumor biology with radiomics: A systematic review utilizing a radiomics quality score. Radiother Oncol 2018;127:349-60. [Crossref] [PubMed]
- Cozzi L, Dinapoli N, Fogliata A, et al. Radiomics based analysis to predict local control and survival in hepatocellular carcinoma patients treated with volumetric modulated arc therapy. BMC Cancer 2017;17:829. [Crossref] [PubMed]
- Nakashima O, Sugihara S, Kage M, et al. Pathomorphologic characteristics of small hepatocellular carcinoma: a special reference to small hepatocellular carcinoma with indistinct margins. Hepatology 1995;22:101-5. [PubMed]
- The International Consensus Group for Hepatocellular Neoplasia. Pathologic diagnosis of early hepatocellular carcinoma: a report of the international consensus group for hepatocellular neoplasia. Hepatology 2009;49:658-64. [Crossref] [PubMed]
- Chen S, Zhu Y, Liu Z, et al. Texture analysis of baseline multiphasic hepatic computed tomography images for the prognosis of single hepatocellular carcinoma after hepatectomy: A retrospective pilot study. Eur J Radiol 2017;90:198-204. [Crossref] [PubMed]
- Zhou Y, He L, Huang Y, et al. CT-based radiomics signature: a potential biomarker for preoperative prediction of early recurrence in hepatocellular carcinoma. Abdom Radiol (NY) 2017;42:1695-704. [Crossref] [PubMed]
Cite this article as: Chang HC, Dai YH, Shen PC, Chang WC, Lo CH, Yang JF, Lin CS, Chao HL, Tu SJ, Huang WY, Hwang JM. Using computed tomography-based radiomics to predict outcomes for hepatocellular carcinoma patients receiving stereotactic body radiotherapy. Ther Radiol Oncol 2021;5:13.