Integrated radiomic model for predicting the prognosis of esophageal squamous cell carcinoma patients undergoing neoadjuvant chemoradiation
Introduction
Esophageal cancer is the fifth leading cause of male cancer mortality in Taiwan, accounting for approximately 1,600 deaths annually (1). In total, 72% of patients are diagnosed at the advance stage. The most common type of esophageal cancer in Asia is differentiated esophageal squamous cell carcinoma (ESCC), presenting different etiology pattern in the occidental world (2). Neoadjuvant concomitant chemoradiation (NACCRT) effectively increases the feasibility of complete surgical resection and thus, has become the standard treatment modality for advanced esophageal cancer in the past 20 years (3,4). Clinical studies on NACCRT for esophageal cancer have reported that pathological complete response (pCR) predicts better disease control and survival outcomes (5). Meanwhile, poor responders show poor prognosis despite adjuvant treatment for disease control (6). However, the decision-making factors for the post-operative adjuvant therapy after NACCRT for ESCC remain not well defined. Undoubtedly, predictive models of response to NACCRT can provide crucial information before surgery.
Computed tomography (CT) is widely used in post-NACCRT surveillance of esophageal cancer. However, it has limitations in terms of distinguishing the residual tumor from the post-treatment effect via visual interpretation (7). Interpreting clinical images using a combination of radiomics and machine learning is a new field in artificial intelligence. Radiomics has been proven to be useful in cancer staging and clinical evaluation (8,9). Texture-based radiomics features are potential robust predictors of clinical outcomes of various cancers (10,11).
For establishing a prediction model of response and prognosis in patients undergoing chemoradiation, there are 2 ways of choosing the objects for extracting radiomics features. One way involves the use of pre-treatment images, which have already been used in numerous studies in recent years. Pre-treatment images are closely correlated to individual differences, helpful in identifying high-risk patient groups, and often used in establishing constrain models following chemoradiation (12-15). The other way involves using the image series taken after chemoradiation. Post-treatment images more closely reflect the treatment effect relationship, such as immune reaction and alteration of the tumor microenvironment. However, high rates of false-positive signals are the major technical problem limiting the application of these images. Radiomics becomes valuable in such situations.
The purpose of this study was to establish a prediction model of pathological response, failure pattern, and survival in advance ESCC patients by calculating texture-based radiomics features of CT images after NACCRT. We hypothesized that radiomics would extract more information from post-chemoradiation CT images and thus, could reduce the treatment effect and focus on the tumor-related factors influencing prognosis.
Methods
Patient demographic data and workup
This retrospective, single-center study evaluated patients with locally advanced esophageal cancer who underwent NACCRT followed by total esophagectomy and gastric tube reconstruction between 2013 and 2018. Those with Eastern Cooperative Oncology Group (ECOG) Performance Status score >1 or biopsy-proven differentiated adenocarcinoma were excluded. Response and outcome were evaluated in 62 patients. Cancer staging was performed using CT, endoscopic ultrasound, esophagogastroduodenoscopy, and whole body 2-deoxy-2-[fluorine-18]-fluoro-D-glucose positron emission tomography integrated with CT (FDG PET/CT). Post-treatment surveillance was assessed 5–8 weeks after completion of NACCRT by performing CT and whole body FDG-PET/CT (optional). Staging was performed according to the TNM classification system (seventh edition) of the American Joint Committee on Cancer. Grade of toxicity was evaluated according to the National Cancer Institute’s Common Terminology Criteria for Adverse Events version four.
Radiotherapy delivery and chemotherapy administration
All eligible patients underwent cancer treatment according to the standard operating procedures at our institution. Supine, contrast-enhanced, alpha cradle® kits (Smithers Medical Products) immobilized CT images were performed for simulation. Simulation images included the entire neck, thorax, and upper abdomen; CT was performed with 3-mm slice thickness and OMAR reconstruction (optional) to avoid metallic shadows. Target volume delineation was performed on the Eclipse platform according to the criteria described below:
- Gross tumor volume (GTV): primary tumor and lymphadenopathy observed on CT and/or 18F-FDG-PET/CT.
- High-dose clinical target volume (CTVHigh): entire GTV with the margins extended 1 cm circumferentially and 3–4 cm longitudinally.
- Low-dose clinical target volume (CTVLow): area of the regional lymphatic nodes if suspected of involvement; for instance, the supraclavicular fossa if the primary tumor or any lymphadenopathy were detected in the upper mediastinum or the celiac lymphatics if the primary tumor or any lymphadenopathy was present close to the esophagogastric junction.
- High-dose and low-dose planning target volume (PTVHigh and PTVLow): addition of CTVHigh and CTVLow with a 0.5-cm expanding margin in all directions.
We delivered 6-MV or 10-MV photon beams via intensity-modulated radiation therapy or volumetric arc therapy (VMAT) planning using the Eclipse treatment planning system version 13 (Varian Medical Systems Inc., Palo Alto, CA, USA) or Pinnacle treatment planning system versions 9.2 and 10.1 (Philips Healthcare Inc., Andover, MA, USA). The PTVHigh and PTVLow were prescribed radiation doses of 48 and 43.2 Gy, respectively, divided into 24 fractions using a simultaneous integrated boost method. The 100% coverage of the prescribed dose volume should exceed 95% of the targeted PTV whilst meeting the normal organ constraints, including the following: maximum dose to the spinal cord should be <45 Gy, volume of the lung receiving >20 Gy should be <30% of the whole lung; and mean dose to the heart should be <30 Gy.
All patients underwent concomitant chemoradiotherapy with the following weekly platinum-based regimens: (I) cisplatin 30 mg/m2; (II) carboplatin (AUC =2); or (III) paclitaxel 50 mg/m2 plus either cisplatin 30 mg/m2 or carboplatin (AUC =2).
Image processing and extraction of radiomics features
Three patients were excluded from image processing: two were excluded because of poor quality of post-treatment CT images due to metal implant and one was excluded because of unfinished radiotherapy. The CT or PET/CT images after NACCRT were imported to the Eclipse treatment planning system (version 13). Planning CT images from 59 patients were rigidly co-registered with post-treatment images according to the gray level inside clipbox, sized in whole chest and centrally aligned in planning center, to avoid unwanted deformation of target organs. The planning GTV were duplicated to its relative post-treatment CT images and defined as regions of interest (ROIs). The ROIs of GTV and relative CT series were inserted into the LIFEx software (16) (version 4.7) to extract radiomics features as follow: 3-dimensional Lagrangian polygon interpolation was applied for resampling images into 1×1×3 mm3 voxels. Hounsfield units (HUs) in all the images were then resampled into 400 discrete values (bins) with absolute discretization from –1,000 to 3,000 HUs. Grey-level matrices were calculated in 3 dimensions to gather 46 radiomic features.
Statistical analysis and computing prediction model
Statistical analysis was performed using the Statistical Package for the Social Sciences for Windows, SPSS® software v. 18.0 (IBM Corp., New York, NY, USA; formerly SPSS Inc., Chicago, IL, USA) and R computing software (version 3.6.0) under the environment of RStudio (version 1.2.1335.) The correlation among pathological complete remission, recurrence pattern, clinical features, and radiomics features were analyzed using the Pearson correlation coefficient (PCC). Clinical features showing significant correlation with complete remission and recurrence patterns were analyzed as predictors using logistic regression. Radiomics feature reduction and regularization of the regression model was performed using the least absolute shrinkage and selection operator (Lasso) formula. Correlation coefficients of Lasso-selected features were calculated and presented as a correlation matrix for reducing collinearity. The predictability of the radiomic features model was assessed by area under the curve (AUC) analysis of the receiver-operating characteristic (ROC) curve. Cut-off points of the expected value in prediction models were assessed by the Youden index (sensitivity + specificity – 1). The Kaplan-Meier method was used to calculate overall survival (OS) and progression-free survival (PFS), which were determined from the date of biopsy to the date of last follow-up. The association of OS and PFS with clinical or radiomics features was analyzed using Cox regression.
Results
Patient characteristics
Table 1 shows the characteristics of the 62 advanced thoracic ESCC patients treated with NACCRT. Median age at diagnosis was 60 years (range, 40–79 years). The majority of patients had clinical stage III disease (n=50, 80.6%), cT3 disease (n=48, 77.4%), lymph node positivity (n=60, 96.8%), and histological grade 2 differentiation (n=43, 69.4%). Primary tumor at the middle esophagus was observed in 51.6% of patients (n=32). Median follow-up duration was 20.6 months (range, 5.2–60.8 months).
Table 1
Characteristic | Case, N (%) |
---|---|
Total | 62 |
Gender (M:F) | 60:2 |
Age | 60.8±8.3 |
Performance status (ECOG score) | |
0 | 39 (62.9) |
1 | 21 (33.9) |
2 | 2 (3.2) |
Clinical T stage | |
T1 | 2 (3.2) |
T2 | 10 (16.1) |
T3 | 48 (77.4) |
T4a | 2 (3.2) |
Clinical N stage | |
N0 | 2 (3.2) |
N1 | 27 (43.5) |
N2 | 24 (38.7) |
N3 | 9 (14.5) |
Clinical M stage | |
M0 | 59 (95.2) |
M1 | 3 (4.8) |
Clinical stage | |
IIB | 9 (14.5) |
IIIA | 22 (35.5) |
IIIB | 17 (27.4) |
IIIC | 11 (17.7) |
IV | 3 (4.8) |
Differential grade | |
NA | 10 (16.1) |
1 | 0 (0.0) |
2 | 43 (69.4) |
3 | 9 (14.5) |
Esophagus position | |
Upper | 14 (22.6) |
Middle | 32 (51.6) |
Lower | 16 (25.8) |
Data are presented as mean ± standard deviation for age and as case number (percentage) for other variables; NACCRT, neoadjuvant concomitant chemoradiation; ECOG score, Eastern Cooperative Oncology Group performance status score; NA, no assessment of the differential grade owing to the quantity of the endoscopy-aided biopsy sample obtained.
Treatment compliance, outcomes, and toxicities
The study cohorts showed favorable tolerance, such that 60 of 62 (96.8%) patients completed both radiotherapy and the entire chemotherapy course. Interruption of NACCRT occurred in one patient who developed intolerable fatigue during treatment. Concomitant chemotherapy was discontinued early in one elderly patient who developed severe nausea and weakness after chemotherapy. The clinical treatment outcomes are summarized in Table 2. The clinical down-staging rate was 77.4%. The pCR rate was 40.3% (25/62). The 1-, 3-, and 5-year OS rates were 74.7%, 48.8%, and 39.8%, respectively. The 1-, 3-, and 5-year PFS rates were 60.1%, 30.6%, and 18.1%, respectively. The local recurrence rate was 19.4% (12/62), and the distant recurrence rate was 37.1% (23/62). Kaplan-Meier survival curves of OS and PFS are shown in Figure 1A,B. In total, 6 patients showed hematological toxicities greater than grade 3. Two patients developed acute radiation pneumonitis ≥ grade 3, one of which died from pneumonitis-induced acute respiratory distress syndrome. One patient died owing to post-surgery infection.
Table 2
Characteristic | Case, N (%) |
---|---|
Concomitant chemotherapy | |
Single platinum | 34 (54.8) |
Platinum-taxol | 26 (41.9) |
Others | 2 (3.2) |
Chemotherapy dose adjustment | |
No | 41 (66.1) |
Yes | 21 (33.9) |
Adjuvant chemotherapy | |
Yes | 31 (50.0) |
No | 31 (50.0) |
Treatment compliance | |
Complete | 60 (96.8) |
Incomplete CT | 1 (1.6) |
Incomplete CT and RT | 1 (1.6) |
Response | |
CR | 24 (38.7) |
PR | 24 (38.7) |
SD | 8 (12.9) |
PD | 6 (9.7) |
Excision status | |
R0 | 58 (93.5) |
R1 | 2 (3.2) |
R2 | 2 (3.2) |
Pathology T stage | |
T0 | 24 (38.7) |
Tis | 1 (1.6) |
T1 | 7 (11.3) |
T2 | 14 (22.6) |
T3 | 15 (24.2) |
T4a | 1 (1.6) |
Pathology N stage | |
N0 | 49 (79.0) |
N1 | 5 (8.1) |
N2 | 6 (9.7) |
N3 | 2 (3.2) |
Data are presented as mean ± standard deviation for treatment duration and case number (percentage) for other variables; weekly platinum, patients underwent weekly cisplatin or weekly carboplatin treatment; NACCRT, neoadjuvant concomitant chemoradiation; CT, chemotherapy; RT, radiotherapy.
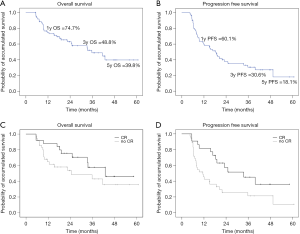
Response and survival predictive capabilities of clinical features
Correlation coefficients of all 25 clinical features for complete response (CR), local regional failure (LRF), and distant failure (DF) were estimated with double-tail PCC analysis. Clinical T stage and anemia were negatively correlated with CR. CR was the only feature that was negatively correlated with LRF. Clinical N stage, overall clinical stage, pathological T and N stages, clinical response, and resection status were correlated with DF. In Kaplan-Meier analysis, patients with and without CR showed significantly different survival curves (Figure 1C,D). Features showed statistical significance of PCC (P<0.05) was further established its log risk model to CR, LRF, and DF by logistic regression (Table 3). Furthermore, the hazard ratios of clinical features predicting PFS and OS were calculated using Cox regression (Table 4). Adjuvant chemotherapy caused a 2.79-fold reduction in mortality risk, whereas an increase in pathology N stage and anemia grade caused a 1.8-fold increase in hazard radio of mortality. Resection status was the strongest positive predictor of the hazard ratio of progression whereas anemia and pT and pN stage moderately predicted the hazard ratio of progression. Treatment response negatively predicted progression, with an expected hazard ratio of 0.6.
Table 3
Features | Beta(x) | SE | P value | Exp. (95% CI) |
---|---|---|---|---|
CR prediction model (logistic regression) | ||||
Clinical T stage | −1.586 | 0.685 | 0.021* | 0.205 (0.054–0.783) |
Anemia | −1.477 | 0.491 | 0.003* | 0.228 (0.087–0.598) |
Constant | 5.810 | 2.257 | 0.010* | – |
Pseudo-R2 =0.345 | ||||
LRF prediction model (logistic regression) | ||||
CR | −1.349 | 0.708 | 0.057 | 0.259 (0.065–1.038) |
Constant | −0.496 | 0.339 | 0.143 | – |
Pseudo-R2 =0.277 | ||||
DF prediction model (logistic regression) | ||||
cN stage | 0.868 | 0.427 | 0.042* | 2.381 (1.031–5.501) |
pT stage | 0.496 | 0.316 | 0.117 | 1.642 (0.884–3.052) |
pN stage | 0.723 | 0.520 | 0.164 | 2.061 (0.744–5.707) |
Response | 0.134 | 0.516 | 0.795 | 1.143 (0.416–3.140) |
Constant | −3.452 | 2.220 | 0.120 | – |
Pseudo-R2 =0.299 |
The expect value of the features was presented through a log risk ratio obtained using logistic regression, followed by the 95% confidence interval. The results of the goodness of fit test were presented as a pseudo-R square value obtained using the Nagelkerke method. *, star marked as statistical significance. Beta(x), coefficient of variate X; SE, standard error of coefficient, Exp., expected value; CI, confidence interval;
Table 4
Features | Beta(x) | SE | P value | Exp. (95% CI) |
---|---|---|---|---|
Significant predictors of OS in the logistic regression | ||||
Adj. CTx | −1.024 | 0.422 | 0.015 | 0.359 (0.157–0.821) |
Anemia | 0.611 | 0.268 | 0.023 | 1.843 (1.089–3.118) |
pN stage | 0.605 | 0.191 | 0.002 | 1.831 (1.259–2.661) |
UHmin | 1.747 | 0.801 | 0.029 | 5.737 (1.195–27.549) |
SHAPE_Sphericity | −1.881 | 0.916 | 0.040 | 0.152 (0.025–0.917) |
GLRLM_GLNU | 1.930 | 0.825 | 0.019 | 6.888 (1.368–34.677) |
GLZLM_LZE | 1.465 | 0.734 | 0.046 | 4.327 (1.026–18.240) |
GLZLM_LZHGE | 1.454 | 0.735 | 0.048 | 4.281 (1.013–18.095) |
PFS prediction model (logistic regression) | ||||
Anemia | 0.669 | 0.219 | 0.002 | 1.953 (1.270–3.002) |
pT stage | 0.294 | 0.111 | 0.008 | 1.341 (1.078–1.669) |
pN stage | 0.468 | 0.182 | 0.010 | 1.597 (1.118–2.282) |
Response | −0.505 | 0.168 | 0.003 | 0.603 (0.434–0.839) |
Resection status | 0.865 | 0.348 | 0.013 | 2.376 (1.201–4.700) |
GLRLM_SRE | −1.585 | 0.786 | 0.044 | 0.205 (0.044–0.955) |
GLRLM_LRE | 1.688 | 0.817 | 0.039 | 5.410 (1.092–26.810) |
GLRLM_RP | −1.667 | 0.804 | 0.038 | 0.189 (0.039–0.914) |
GLZLM_LZE | 1.429 | 0.696 | 0.040 | 4.175 (1.067–16.340) |
GLZLM_LZHGE | 1.393 | 0.702 | 0.047 | 4.026 (1.018–15.924) |
The expected value of the features was presented as the hazard ratio followed by the 95% confidence interval. The goodness of fit test is presented using the omnibus test. Beta(x), coefficient of variate X; SE, standard error of coefficient, Exp., expected value; CI, confidence interval.
Response- and survival-related predictive capabilities of radiomics features
Eight features [1 shape feature, 1 neighborhood grey-level different matrix (NGLDM) feature, 2 grey-level co-occurrence matrix (GLCM) features, and 4 grey-level zone length matrix (GLZLM) features], including PCC (Figure 2A) were selected using the Lasso regularization formula (Figure 2B). The regression model comprising the abovementioned 8 features showed good capability with acceptable representativeness (AUC =0.851, pseudo-R2 =0.504; ROC curve presented in Figure 2C) for predicting CR to NACCRT. The prediction model of LRF and DF with features restricted by the Lasso formula (left panel of Figure 3) had medium capability but poor representativeness (AUC =0.773 and 0.721, pseudo-R2 =0.291 and 0.213, respectively; ROC curve presented in the right panel of Figure 3). Using K-fold cross validation by the glmnet package of R software, radiomics features having unreliable coefficients were first excluded (Figure S1A,B). The Cox regression model showed that minimal UH, GLRLM_GLNU, GLZLM_LZE, and GLZLM_LZHGE strongly increased the hazard ratio of mortality by 4- to 6-folds, whereas sphericity of shape strongly reduced the hazard ratio of mortality by 6.6-folds. For PFS, 3 GLRLM family and 2 GLZLM family radiomics strongly predicted the hazard ratio of progression.
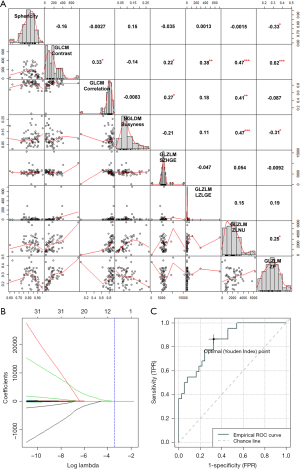
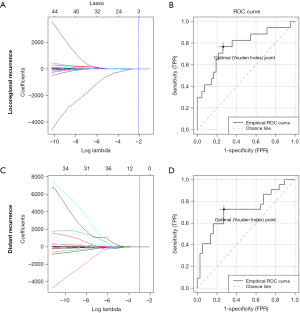
Combining clinical and radiomics features for enhancing prediction power and reliability
Individually, clinical and radiomics features had problems in terms of prediction power and reliability for predicting LRF and DF. We combined both clinical and radiomic features to develop a new prediction model. In the LRF prediction model that combined the clinical feature “complete response” and 3 radiomics features, the AUC was 0.804 (Figure 4A) and pseudo-R square was 0.339. Contrastingly, in the DF prediction model that combined clinical and radiomic features, the AUC and pseudo-R square values were 0.754 (Figure 4B) and 0.320, respectively. The formula of LRF and DF prediction models using a combination of clinical and radiomic features were detail in Table 5. Both the LRF and DF prediction models with combined clinical and radiomic features had stronger power and better reliability than those using each type of feature individually. Because of the limitation of censored numbers, Cox regression could only proceed with one variable in the overall prediction model in the present study. Therefore, we only tested the combination of one clinical feature and one radiomics feature in the prediction model of PFS. The combination of resection status and selected radiomics, any of the 5 radiomic predictors having significant correlation to hazard ratio of progression, mutually enhanced the expected hazard ratio value of progression. The strongest combination was resection status multiplied by GLRLM_LRE, which resulted in an expected hazard ratio value of progression of 8.776 (95% CI: 1.959–39.320, P=0.005) and good model reliability, tested using the chi-square test of residuals and the omnibus test.
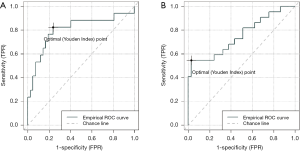
Table 5
Features | Beta(x) | SE | P value | Exp. (95% CI) |
---|---|---|---|---|
LRF prediction model (logistic regression) | ||||
Complete response | −1.274 | 0.857 | 0.137 | 0.280 (0.052–1.499) |
SHAPE_Sphericity | −3.104 | 1.829 | 0.090 | 0.045 (0.001–1.619) |
NGLDM_Busyness | 4.017 | 1.702 | 0.018* | 55.533 (1.977–1.560×103) |
GLZLM_LZLGE | 2.840 | 2.264 | 0.210 | 17.110 (0.202–1.447×103) |
Constant | −0.119 | 1.193 | 0.921 | – |
Pseudo-R2 =0.339 | ||||
DF prediction model (logistic regression) | ||||
pN stage | 0.954 | 0.475 | 0.045* | 2.595 (1.022–6.590) |
SHAPE_Sphericity | −4.387 | 1.963 | 0.025* | 0.012 (0.000–0.583) |
GLRLM_LRE | −1.378 | 3.341 | 0.680 | 0.252 (0.000–1.760×102) |
GLRLM_GLNU | −1.063 | 2.124 | 0.617 | 0.345 (0.005–2.222×101) |
GLZLM_LZLGE | 3.490 | 2.485 | 0.160 | 32.780 (0.252–4.271×103) |
GLZLM_ZP | −4.957 | 3.122 | 0.112 | 0.007 (0.000–3.200) |
Constant | 4.405 | 2.874 | 0.125 | – |
Pseudo-R2 =0.320 |
The expect value of the features was presented through a log risk ratio obtained using logistic regression, followed by the 95% confidence interval. The results of the goodness of fit test were presented as a pseudo-R square value obtained using the Nagelkerke method. *, star marked as statistical significance. Beta(x), coefficient of variate X; SE, standard error of coefficient, Exp., expected value; CI, confidence interval.
Discussion
The current esophageal cancer treatment guidelines recommend CT or PET/CT as the standard of response assessment (4). However, even after 4–6-week recovery intervals, residual tumors at the esophagus on contrast-enhanced CT could be masked by submucosal swelling via visual interpretation (12). It is also difficult to differentiate residual lymph nodes at the pulmonary hilum with the bare human eye (17). Endoscopic ultrasound and biopsy can hardly detect submucosal residual tumors, which are suggested to be predictors of poor pathological outcomes (18). Post-NACCRT surveillance generally involves FDG-PET/CT; although FDG-PET/CT is good in differentiating residual lymph nodes, they tend to get obscured by treatment-induced inflammation (12). A good post-treatment surveillance method is yet to be developed.
Radiomics, known as the next generation technology of computer-aided diagnosis and detection systems, is more capable than answering yes-or-no questions in lesion detection. With the combination of texture extraction, artificial intelligence, and new algorithms that are able to reduce variates, radiomics can not only be applied in disease diagnosis but also used to extract more information for helping clinical decision making (19). A good example is the application of radiomics in treatment response evaluation after neoadjuvant treatment for nasopharyngeal cancer (NPC) and rectal cancer (20,21); with this technique, the surgeon has more information before performing the operation. Recent studies have also evaluated radiomics characteristics for directly predicting cancer prognosis, for instance in lung cancer, NPC, and hepatic cancer (10,11,13).
In 2016, van Rossum reviewed the evidence and potential future of applying radiomics in the field of esophageal cancer (22). By using pre-treatment contrast-enhanced CT, Hou et al.’s study suggested that radiomics could predict treatment response to definitive chemoradiation in inoperable esophageal cancer patients (23). However, Hou et al.’s study had different predictors to PD, SD, PR, and CR, indicating that they did not have a unified prediction model for treatment response. Jin et al.’ study used a combination of radiotherapy dosimetry and radiomics to predict chemoradiation treatment response (14) and integrated 63 features in one prediction model. However, many of these features showed strong linear correlation in the Person correlation matrix. More than 2 studies did not have good prediction power (AUC =0.6–0.7) and did not mention evidence of robustness and reliability. Larue et al.’s study focused on esophageal cancer patients who underwent NACCRT and directly predicted 3-year OS using a model based on radiomics features extracted from pre-treatment CT images (15). However, Larue et al.’s model reported an AUC of 0.61 in the validation dataset, and the risk group predicted by the model showed no significant correlation with pathological response, which is not consistent with the current clinical consensus.
According to our review of the literature, this is the first study in esophageal cancer research that attempted to establish a prediction model based on post-NACCRT CT images. With regards to prediction models of pCR and failure patterns, radiomics showed better capability and representativeness than that seen in previous studies based on pre-treatment CT images. LIFEx, a widely accepted freeware with literature support was used to extract 42 histogram, textural and shape radiomics features. As the result (Figure 1C,D), significantly longer PFS and OS were observed in the pCR group than in the non-pCR group. Therefore, our high prediction power model showed in Figure 2 is useful for deciding between esophagectomy and definitive chemoradiation. Furthermore, in the high-risk patient group, the failure pattern prediction model indicated that adjuvant treatment after surgery might have prevented local or distant recurrence.
A few clinical and radiomic predictors were common to the prediction models of DF pattern, PFS, and OS. Residual pathological lymph node significantly affected prognosis, as observed in this study and widely discussed in other studies (6). In the Cox regression model for predicting PFS and OS, radiomics features showed more power for predicting hazard ratios than clinical features. Moreover, both failure pattern and survival prediction models with combined clinical and radiomic predictors showed synergistically enhanced prediction powers. Interestingly, pathological response was a strong predictor of PFS but not OS. This may indicate that, in addition to residual tumors, other factors in the surrounding environment have an influence on patient survival; the adverse effects of chemoradiation as well as immune response may influence patient survival.
Interpreting the meaning of radiomics may reveal its role in the prediction model. The most important radiomics feature in all prediction models was the long-zone high gray-level emphasis (GLZLM_LZHGE), defined as the distribution of the long homogeneous zones with high grey-levels, representing poor prognosis with a 4-fold increase in hazard ratios of progression and mortality. High sphericity was a negative predictor of LRF, DF, and mortality. Long homogeneous runs in an image (GLRLM_LRE) seem like spiculated extended structures that predict DF and progression. Non-uniformity of the grey-levels (GLRLM_GLNU) is a strong positive predictor of DF and mortality.
The limitation of this study is that the number of patients was too small for separation into the training and validation datasets. Therefore, we were unable to prove the robustness of the prediction model. Furthermore, the retrospective and single-center nature of the study may have introduced selection bias. The established model is still in the preliminary stage. In the future, we aim to test our prediction model by validating the images from the public patient database. We would also improve the algorithm to enhance the accuracy of feature elimination and power of prediction.
In conclusion, we established an integrated model that combined clinical features and post-treatment CT radiomics features for predicting response and survival outcomes of esophageal cancer patients undergoing neoadjuvant chemoradiation. The integrated prediction model may aid clinicians in decision making regarding post-operative adjuvant therapy for ESCC patients who have received neoadjuvant chemoradiation.

Acknowledgments
Funding: This study was funded by MacKay Memorial Hospital (grant number: MMH-E-107-13) and the Ministry of Science and Technology, Taiwan (grant number: MOST 106-2314-B-195-002-MY3 and MOST-106-2623-E-195-001-NU).
Footnote
Conflicts of Interest: The authors have no conflicts of interest to declare.
Ethical Statement: The authors are accountable for all aspects of the work in ensuring that questions related to the accuracy or integrity of any part of the work are appropriately investigated and resolved. The study protocol was granted institutional review board approval (serial number: 18MMHIS194e). Written informed consent was obtained from all study participants and research was conducted in accordance with the 1975 Declaration of Helsinki, as revised in 2013.
Open Access Statement: This is an Open Access article distributed in accordance with the Creative Commons Attribution-NonCommercial-NoDerivs 4.0 International License (CC BY-NC-ND 4.0), which permits the non-commercial replication and distribution of the article with the strict proviso that no changes or edits are made and the original work is properly cited (including links to both the formal publication through the relevant DOI and the license). See: https://creativecommons.org/licenses/by-nc-nd/4.0/.
References
- Health Promotion Administration, Ministry of Health and Welfare, Taiwan (R.O.C). Taiwan Cancer Registry Annual Report, 2016. Available online: https://www.hpa.gov.tw/Pages/Detail.aspx?nodeid=269&pid=10227
- Domper Arnal MJ, Ferrandez Arenas A, Lanas Arbeloa A. Esophageal cancer: Risk factors, screening and endoscopic treatment in Western and Eastern countries. World J Gastroenterol 2015;21:7933-43. [Crossref] [PubMed]
- Lordick F, Mariette C, Haustermans K, et al. Oesophageal cancer: ESMO Clinical Practice Guidelines for diagnosis, treatment and follow-up. Ann Oncol 2016;27:v50-7. [Crossref] [PubMed]
- Network TNCC. NCCN Clinical Practice Guidelines in Oncology (NCCN guidelines) - Esophageal and Esophagogastric Junction Cancers. 2018. (R.O.C). Taiwan Cancer Registry Annual Report, 2016. https://www.nccn.org/professionals/physician_gls/pdf/esophageal.pdf
- Donahue JM, Nichols FC, Li Z, et al. Complete pathologic response after neoadjuvant chemoradiotherapy for esophageal cancer is associated with enhanced survival. Ann Thorac Surg 2009;87:392-8; discussion 398-9. [Crossref] [PubMed]
- Depypere LP, Vervloet G, Lerut T, et al. ypT0N+: the unusual patient with pathological complete tumor response but with residual lymph node disease after neoadjuvant chemoradiation for esophageal cancer, what's up? J Thorac Dis 2018;10:2771-8. [Crossref] [PubMed]
- Sultan R, Haider Z, Chawla TU. Diagnostic accuracy of CT scan in staging resectable esophageal cancer. J Pak Med Assoc 2016;66:90-2. [PubMed]
- Cheng L, Wu L, Chen S, et al. CT-based radiomics analysis for evaluating the differentiation degree of esophageal squamous carcinoma. Zhong Nan Da Xue Xue Bao Yi Xue Ban 2019;44:251-6. [PubMed]
- Wu L, Wang C, Tan X, et al. Radiomics approach for preoperative identification of stages I-II and III-IV of esophageal cancer. Chin J Cancer Res 2018;30:396-405. [Crossref] [PubMed]
- Lv W, Yuan Q, Wang Q, et al. Radiomics Analysis of PET and CT Components of PET/CT Imaging Integrated with Clinical Parameters: Application to Prognosis for Nasopharyngeal Carcinoma. Mol Imaging Biol 2019; [Epub ahead of print]. [Crossref] [PubMed]
- Zhang Y, Oikonomou A, Wong A, et al. Radiomics-based Prognosis Analysis for Non-Small Cell Lung Cancer. Sci Rep 2017;7:46349. [Crossref] [PubMed]
- Borggreve AS, Mook S, Verheij M, et al. Preoperative image-guided identification of response to neoadjuvant chemoradiotherapy in esophageal cancer (PRIDE): a multicenter observational study. BMC Cancer 2018;18:1006. [Crossref] [PubMed]
- Akai H, Yasaka K, Kunimatsu A, et al. Predicting prognosis of resected hepatocellular carcinoma by radiomics analysis with random survival forest. Diagn Interv Imaging 2018;99:643-51. [Crossref] [PubMed]
- Jin X, Zheng X, Chen D, et al. Prediction of response after chemoradiation for esophageal cancer using a combination of dosimetry and CT radiomics. Eur Radiol 2019; [Crossref] [PubMed]
- Larue RT, Klaassen R, Jochems A, et al. Pre-treatment CT radiomics to predict 3-year overall survival following chemoradiotherapy of esophageal cancer. Acta Oncol 2018;57:1475-81. [Crossref] [PubMed]
- Nioche C, Orlhac F, Boughdad S, et al. LIFEx: A Freeware for Radiomic Feature Calculation in Multimodality Imaging to Accelerate Advances in the Characterization of Tumor Heterogeneity. Cancer Res 2018;78:4786-9. [Crossref] [PubMed]
- Udoji TN, Phillips GS, Berkowitz EA, et al. Mediastinal and Hilar Lymph Node Measurements. Comparison of Multidetector-Row Computed Tomography and Endobronchial Ultrasound. Ann Am Thorac Soc 2015;12:914-20. [Crossref] [PubMed]
- Schneider PM, Metzger R, Schaefer H, et al. Response evaluation by endoscopy, rebiopsy, and endoscopic ultrasound does not accurately predict histopathologic regression after neoadjuvant chemoradiation for esophageal cancer. Ann Surg 2008;248:902-8. [Crossref] [PubMed]
- Gillies RJ, Kinahan PE, Hricak H. Radiomics: Images Are More than Pictures, They Are Data. Radiology 2016;278:563-77. [Crossref] [PubMed]
- Liu Z, Zhang XY, Shi YJ, et al. Radiomics Analysis for Evaluation of Pathological Complete Response to Neoadjuvant Chemoradiotherapy in Locally Advanced Rectal Cancer. Clin Cancer Res 2017;23:7253-62. [Crossref] [PubMed]
- Wang G, He L, Yuan C, et al. Pretreatment MR imaging radiomics signatures for response prediction to induction chemotherapy in patients with nasopharyngeal carcinoma. Eur J Radiol 2018;98:100-6. [Crossref] [PubMed]
- van Rossum PSN, Xu C, Fried DV, et al. The emerging field of radiomics in esophageal cancer: current evidence and future potential. Transl Cancer Res 2016;5:410-23. [Crossref] [PubMed]
- Hou Z, Ren W, Li S, et al. Radiomic analysis in contrast-enhanced CT: predict treatment response to chemoradiotherapy in esophageal carcinoma. Oncotarget 2017;8:104444-54. [Crossref] [PubMed]
Cite this article as: Hou TC, Huang WC, Tai HC, Chen YJ. Integrated radiomic model for predicting the prognosis of esophageal squamous cell carcinoma patients undergoing neoadjuvant chemoradiation. Ther Radiol Oncol 2019;3:28.